Analytical imaging in pharma
Boost your clinical trials and drug development with customized analytical imaging in pharma powered by AI. We have experience in creating advanced technology tailored to the specific requirements of pharmaceutical companies.
Our team of experts utilizes both machine and deep learning to develop personalized algorithms for data analysis that reveal the hidden potential of your medical imaging data, extracting valuable insights to expedite your projects.
Enhance your project using analytical imaging in pharma
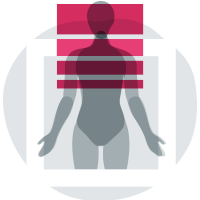
AI in diagnostic imaging
By providing precise measures of drug efficacy and safety, AI in diagnostic imaging has the potential to accelerate the drug development process. We combine both machine and deep learning technology and also experience in working with clinical experts. AI-boost analysis of image data enables you to gain smarter insights and make more informed decisions.
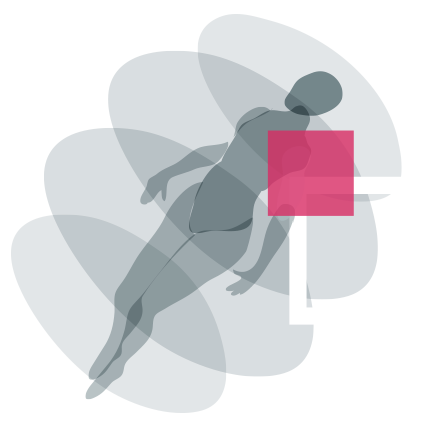
Video & image analysis
In our projects related to pharma, we always take advantage of novel image and video processing techniques to enhance, interpret, translate, segment and analyze medical data. Moreover, our scientific computing team works on the best possible performance of our solutions combining convolutional neural networks, generative adversarial networks (GANs), and computer vision algorithms.
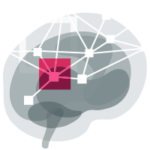
Image analysis in clinical trials
AI has shown great potential in the field of oncology image analysis. What’s more, we have successfully implemented solutions that identify, measure and track lesions across time points. Leverage the experience of our data engineers and ML experts, and maximize the value of the medical image analysis data in your pharma research and early development.
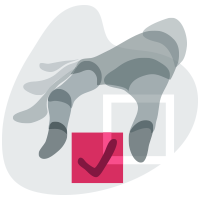
Imaging biomarkers
Quantitative measurements from medical images can provide valuable additional information to support clinical trials and the drug development process. Our experts have experience working with clinical research teams on clinical datasets and also dataset samples to identify imaging features. In summary, translational biomarkers can aid in the drug development process.
Go or no-go decisions
AI-assisted models in medical imaging can speed up the making of decisions in clinical trials by quickly analyzing imaging data. Advanced algorithms can rapidly process vast amounts of medical images, extracting valuable insights that would be time consuming. As a result, having additional data can lead to informed decisions about the next steps in a drug’s development process.
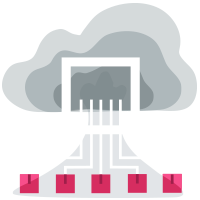
Radiomics for pharma
Radiomics extracts quantitative data from medical images like CT, MRI, and PET scans. This data reveals patterns that can support the clinical trials. By analyzing features like shape or volume, radiomics offers deeper insights into treatment response and disease progress. This method provides access to supplementary information at the microstructural level.
Early development and clinical trials
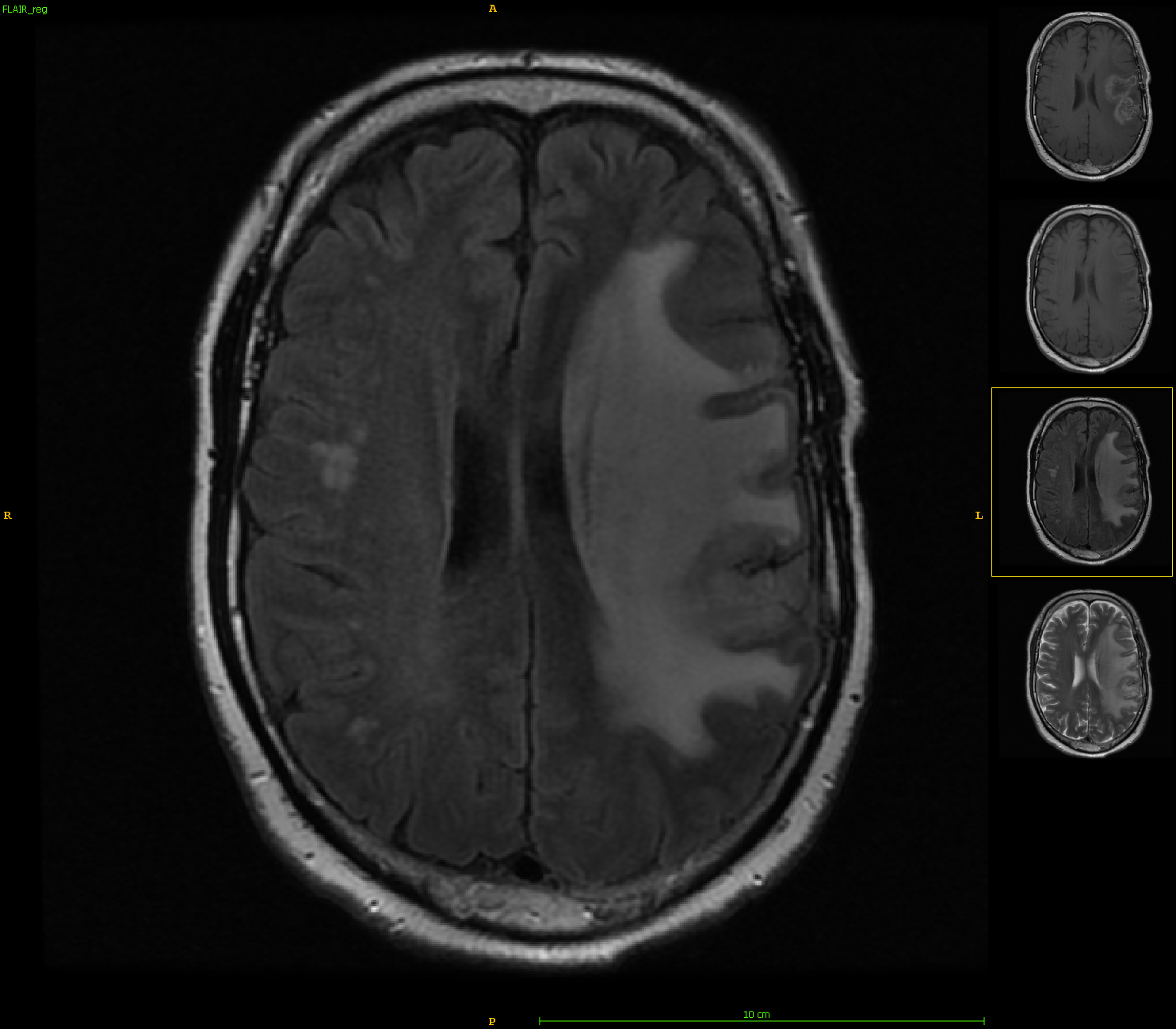
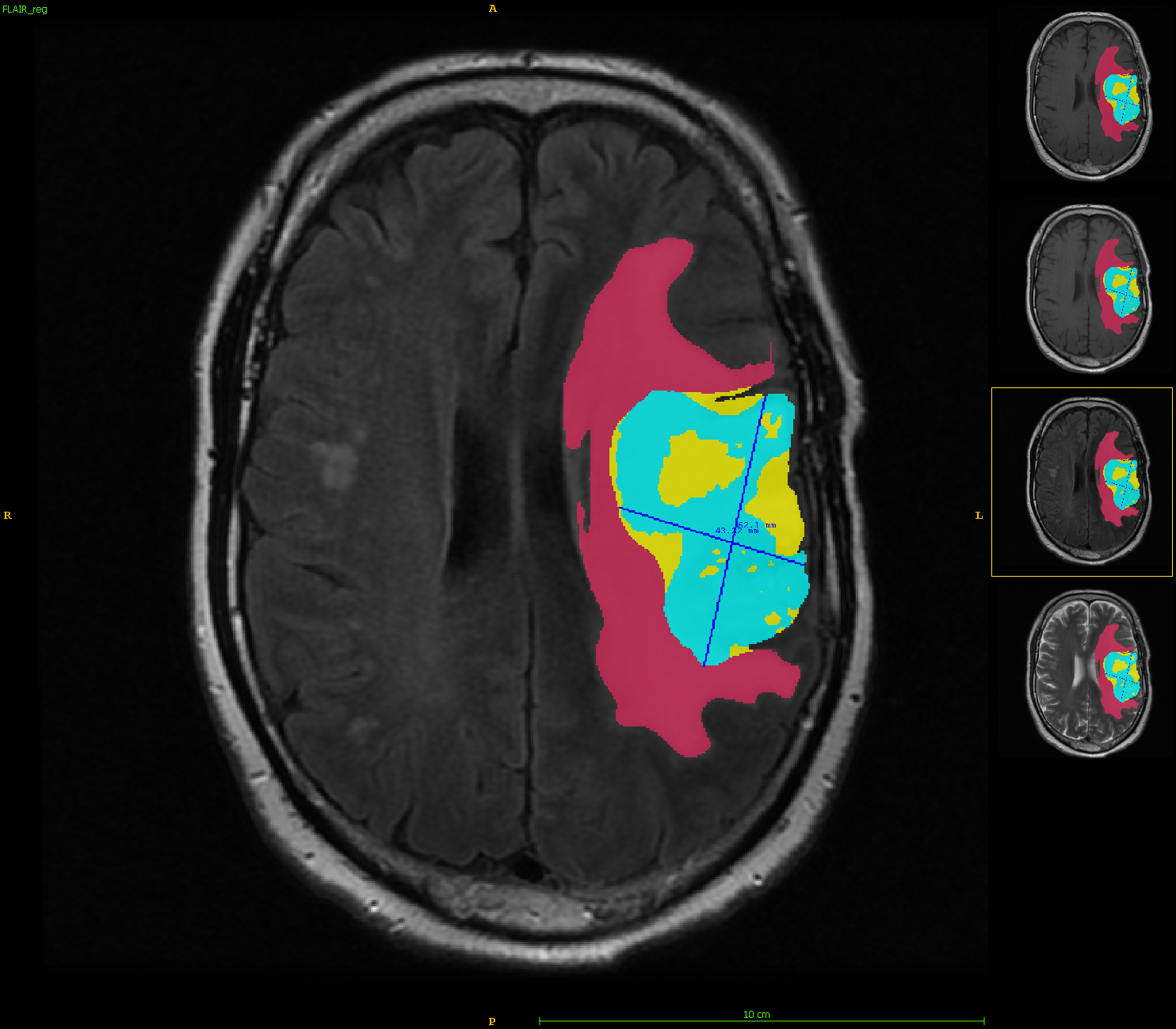
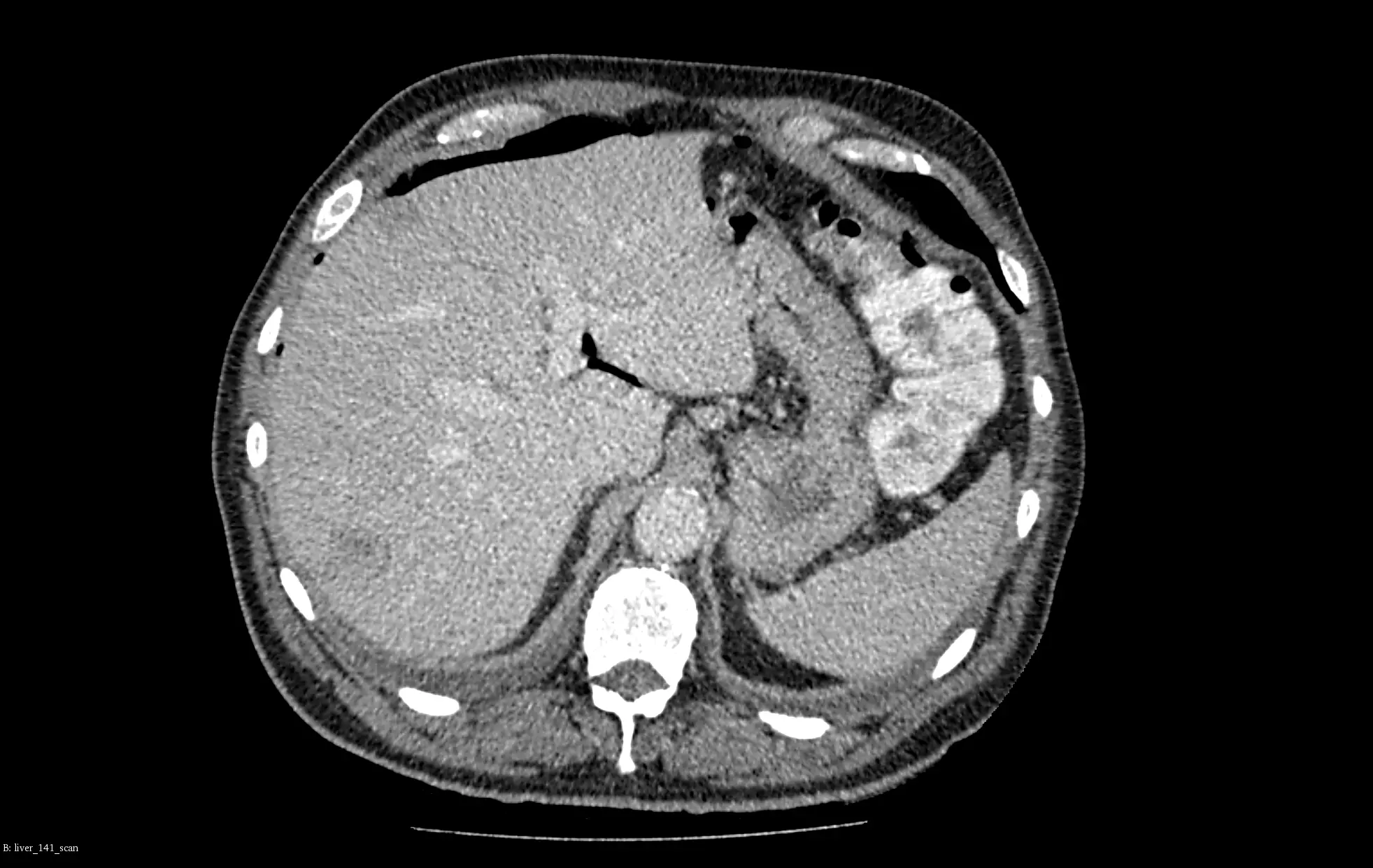
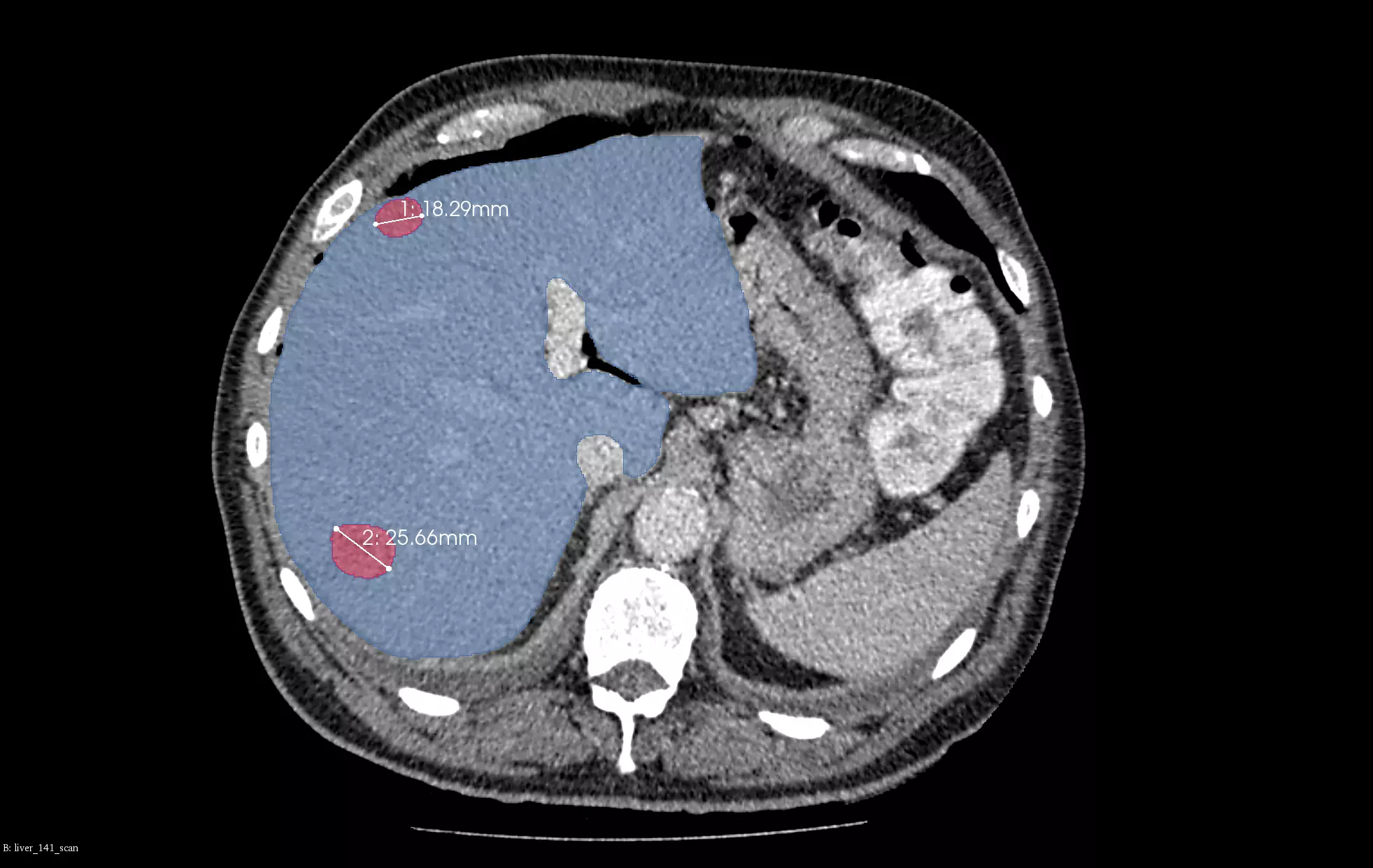
AI in early development phase
Machine learning and AI-enabled image analysis in drug development and the early development phase have the potential to significantly improve and simplify radiological evaluation.
The example above, showing the before and after images, demonstrates our models’ ability to automatically segment brain tumors and identify 3 tumor subregions (edema, enhancing tumor, and necrosis). Additionally, the models provide volume and RANO (Response assessment in neuro-oncology) measurements, making them a valuable tool for monitoring patients in clinical studies.
The statistical power of medical data
Artificial intelligence can enhance the statistical power of medical data by reducing interobserver variability and improving reproducibility.
In the above example, we present our models for liver and liver tumor segmentation. The image shows primary tumors, secondary tumors and metastases. It is based on portal venous CT scans. For clarity, only the two biggest lesions are marked in the visualizations.
Accelerating drug development with imaging biomarkers
Machine learning supports the acceleration of drug development. It helps in creating imaging biomarkers to aid clinical trials. ML models analyze complex imaging data to detect patterns and identify biomarkers. This provides valuable insights into disease progress, drug efficacy, and safety. This enables non-invasive monitoring of therapeutic responses.
Additionally, the FDA emphasizes the important role of translational biomarkers in streamlining drug development—imaging biomarkers associated with higher rates of drug approvals.
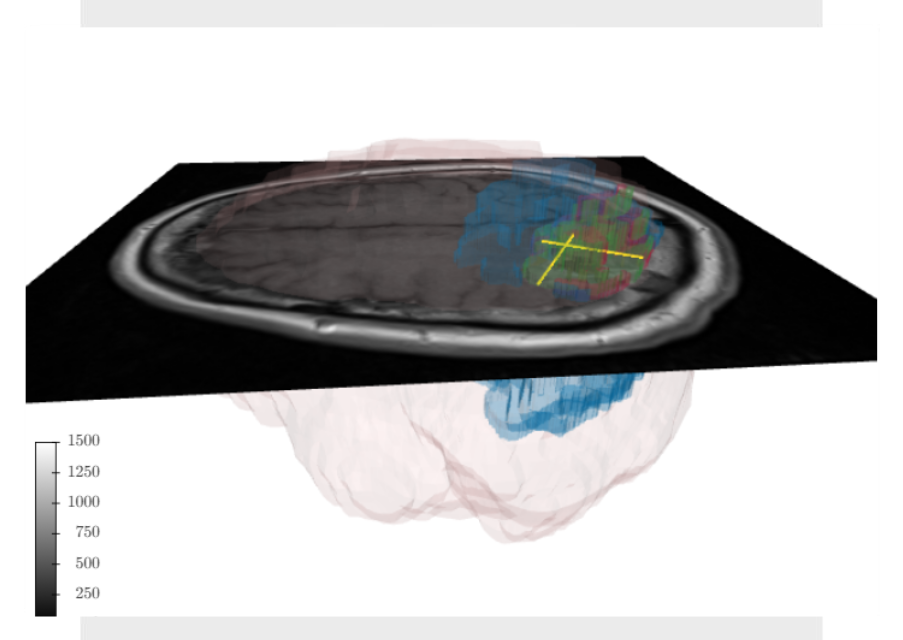
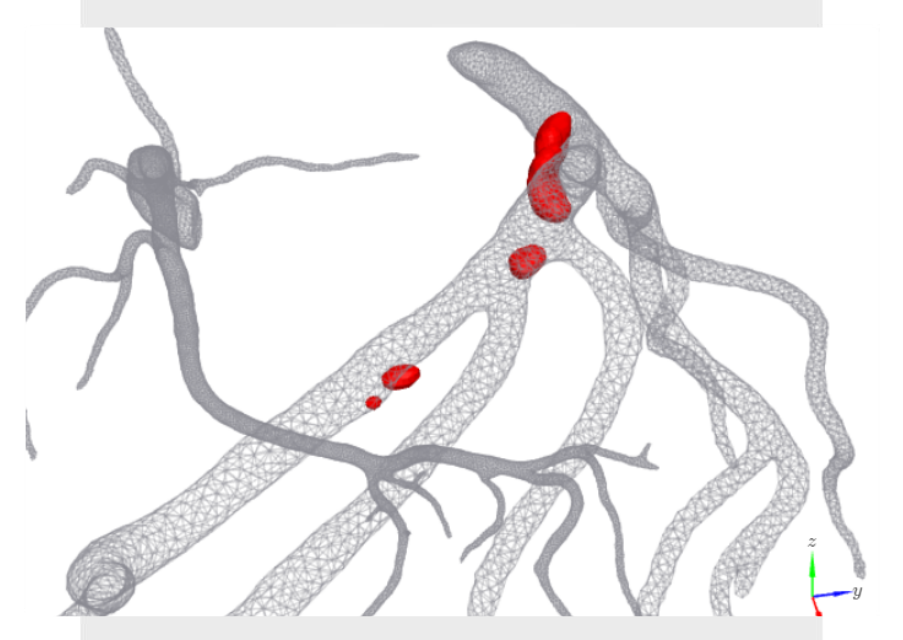
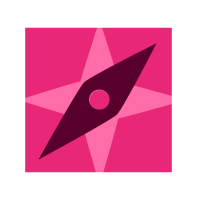
Medical diagnostic video analysis
Artificial Intelligence is revolutionizing drug development by redefining how medical data is analyzed and used. Beyond its traditional applications in analyzing DICOM images from CT, MRI, and other radiological scans, AI is now being harnessed for medical videos, like those from endoscopy procedures. This opens up a world of new possibilities for data analysis.
We combine advanced methods such as machine learning, deep learning, radiomics, convolutional neural networks (CNNs), and generative adversarial networks (GANs) to address complex challenges in medical imaging. These tools help us extract precise image features and generate realistic synthetic data to improve diagnostics and research.
Furthermore, we are actively engaged in virtual non contrast imaging, which produces clear images without contrast agents, thus enhancing safety and efficiency, especially in drug development.
Learn more about our projects for Pharma
In the below paper, we addressed the problem of detection of cirrhosis from CT, which depends on user and lacks reproducibility, and proposed an AI-enabled, reproducible comprehensive approach. Additionally, we showed that selecting the most discriminative features leads to the Pareto-optimal models with enhanced feature-level interpretability as the number of features was dramatically reduced (280) from thousands to tens.
The high performance of our algorithms is well presented in the above paper. Quantitative, qualitative, and statistical analysis revealed that our pipeline performs accurate segmentation of pre and post-operative MRIs in a fraction of the manual delineation time (up to 20 times faster than humans). Additionally, we showed that RANO measurements are not always sufficient to quantify tumor burden and developed a new method of calculating RANO that is more resistant to poor automated segmentations and jagged contouring.